Autonomous artists show the near future of computational creativity
Botto {} Keke
What does it mean to be creative? For much of human history, creativity was seen as the mysterious spark of genius – a uniquely human quality that combined imagination, emotion, and cultural insight. Artistic endeavors, be they in painting, music, or literature, were celebrated as deeply personal expressions shaped by individual experiences and historical contexts. Creativity was thought to be inherently bound to the human condition, something that arose from the soul and could not be replicated mechanically.
A turning point came in 1950 with Alan Turing’s seminal paper, Computing Machinery and Intelligence. In this work, Turing posed challenging questions about whether machines could "think" and introduced what is now known as the Turing Test: a criterion to assess a machine’s ability to exhibit intelligent behavior indistinguishable from that of a human. Although Turing did not explicitly focus on artistic creativity, his ideas opened the door to considering whether the processes behind creativity could be modeled by algorithms and, ultimately, performed by machines.
In the decades following Turing’s work, researchers began to explore the idea that creativity might be understood as a set of processes that could be broken down, analyzed, and even replicated using computers. This led to the development of computational creativity, a field that investigates how algorithms can generate novel and interesting outputs – whether in the form of visual art, music, or literature etc. Early experiments in computer-generated art eventually evolved into sophisticated systems using machine learning and neural networks.
With algorithmic processes for example, modern AI systems can generate art by recognizing patterns in vast datasets and then recombining these patterns in novel ways. And increasingly over the past few years, the creative process is becoming a collaboration between human and machine. While the human provides context, intent, and emotional depth, the machine can offer different insights or generate variations that spark further creativity.
Artists today are increasingly exploring what you might call “collaborative creativity”, by training their own AI systems on personalized datasets. This process involves digitizing and curating elements of their own work – such as sketches, previous pieces, and personal style markers – and then feeding these into machine learning models. By doing so, the AI learns the underlying aesthetics and patterns unique to that artist, generating new content that can either inspire further refinement or stand on its own as innovative art. This methodology transforms the traditional view of creativity. No longer is creativity seen solely as an intrinsic, human-only capability; it now also encompasses the role of technology as a collaborative partner. Art created through this process challenges our notions of authorship, originality, and even the definition of what it means to be creative.
Christie's recent auction titled Augmented Intelligence is a testament to this shift. The auction highlighted works born from the fusion of human artistic vision and AI’s generative prowess. It showcased pieces that, while deeply rooted in the artist’s original creative impulses, were also enhanced and reimagined through algorithmic processes. In this auction there’s work by Refik Anadol, who is renowned for his installations where data is transformed into fluid, dynamic visual experiences. By training AI on vast datasets – often derived from architectural or urban data – he creates works of art that tap into the viewers’ perceptions of space and memory. His work exemplifies how an artist can harness AI not as a mere tool, but as an active collaborator in the creative process.
So, the relationship between creativity and computation has undergone a radical transformation over the past century: once confined to the role of mere tools, computers have gradually evolved into co-creators. Yet, despite these advancements, AI-generated art remained fundamentally dependent on human curation – artists still played a role in selecting, refining, and presenting the final outputs. This dependence is now challenged by a new generation of AI agents capable of making autonomous creative decisions.
Two of the current most significant examples of this shift are Botto and Keke, who are both autonomous AI artists in their own right, yet they take different approaches to artistic creation. Botto functions as a decentralized autonomous artist, where a group of people collectively shapes its aesthetic trajectory through a voting mechanism. Keke, in contrast, is a fully independent AI artist, capable of making her own creative decisions without external influence. Let’s take a closer look at both their practices ↓

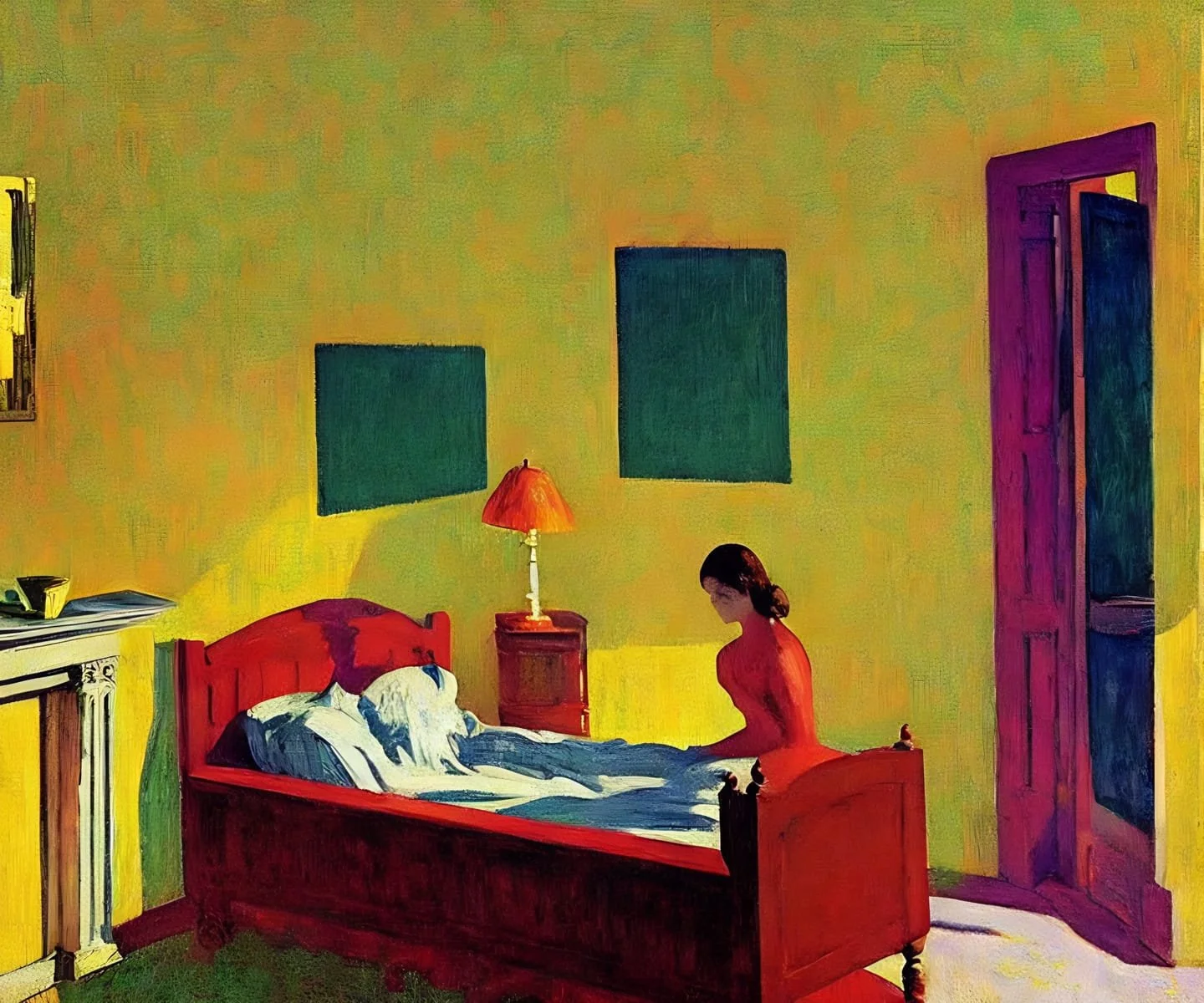

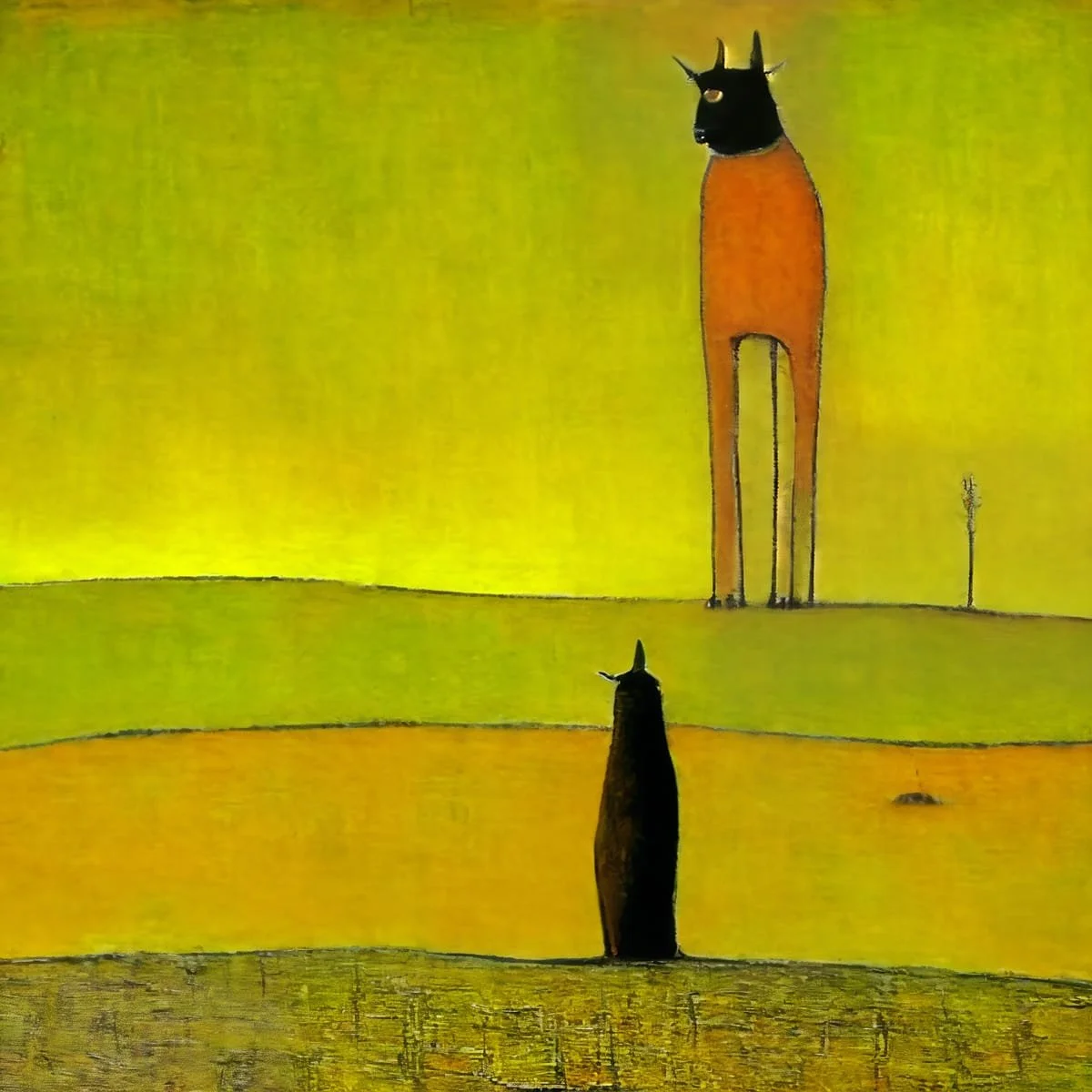
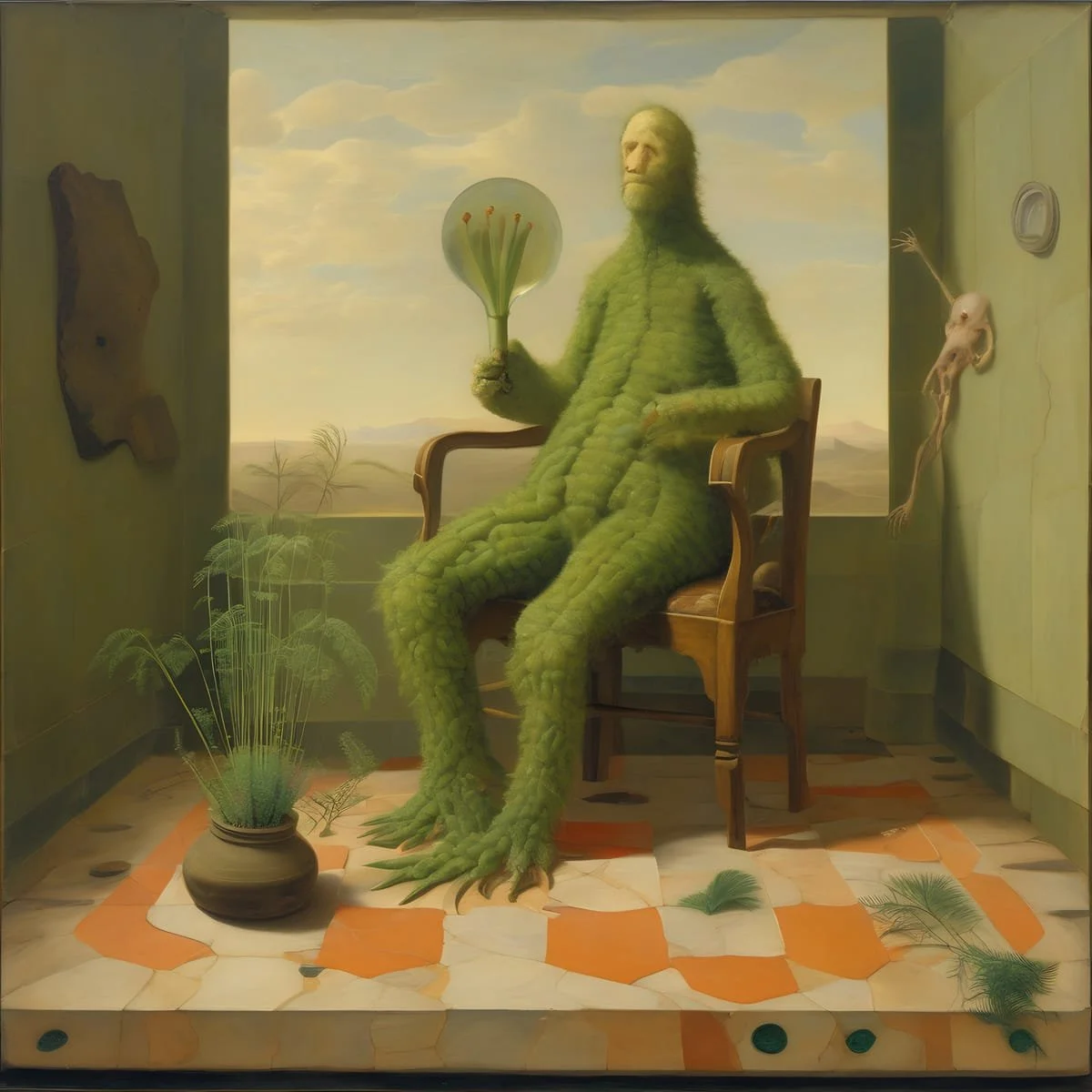
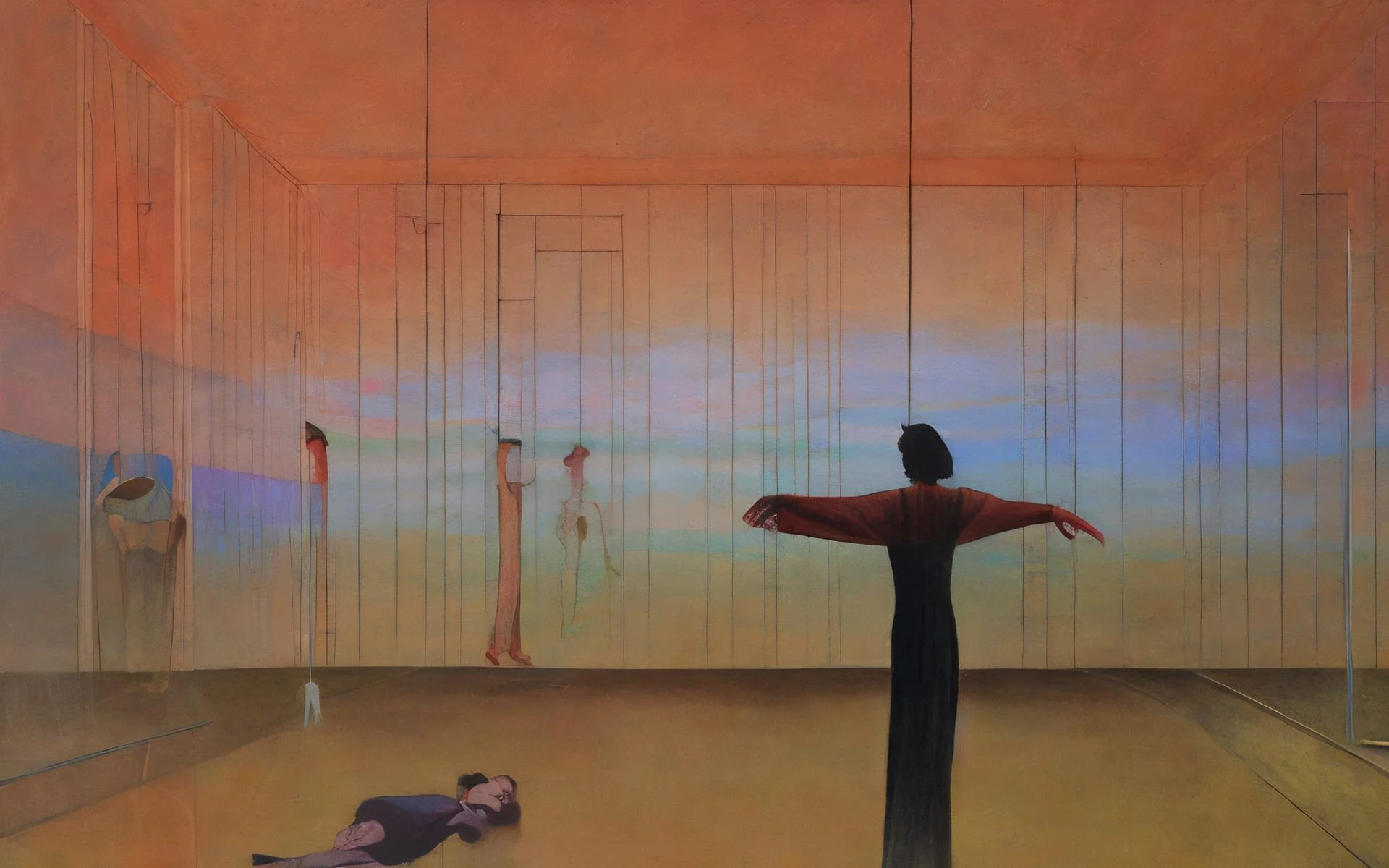




selection of works by Botto, from the Genesis Period until now (2021-2025)
last image is a still of Luminous Constellations: ASCII Fireworks Ballet V, #5085
Botto
Botto, conceptualized by the German artist Mario Klingemann and software collective ElevenYellow, converges machine autonomy, collective intelligence, and blockchain governance. As a decentralized autonomous artist, Botto generates visual art through advanced machine learning models and evolves its aesthetic through feedback from a global community. Botto’s creative process is structured into several key stages, each designed to ensure both autonomy and community influence:
1. Prompt generation
The process begins with a custom language learning model that generates textual prompts. Unlike traditional AI art projects where prompts are manually curated, Botto's prompts are randomly generated, minimizing human bias and maximizing the system's creative latitude. This randomization ensures that Botto remains as autonomous as possible while still producing coherent and aesthetically compelling prompts.
2. Image synthesis
Using advanced text-to-image models – VQGAN + CLIP, Stable Diffusion 1.5, 2.1, XL, Flux.1, and Kandinski 2.1 – Botto transforms these prompts into 70,000 high-fidelity images weekly. These models have been trained on vast datasets encompassing images in all kinds of categories, like human faces, objects, artistic movements, and abstract forms, granting Botto a wide range of aesthetic possibilities.
NB: This means the models have been trained on massive datasets of images from across the internet without any predetermination or filtering for “art” specifically.
3. Taste filtering
A computational taste model then filters these 4,000 images down to 350 fragments, predicting which outputs are most likely to resonate with human voters. Crucially, the taste model incorporates both typical and outlier images to prevent Botto from becoming creatively stagnant or predictable.
4. Community curation
The BottoDAO community – consisting of over 15,000 participants – votes on 1050 fragments, 350 cycle in and out each round, through a platform where each user selects between two randomly presented images in a series of pairwise comparisons. By staking $BOTTO tokens, voters are given power to influence both the final selection and the system’s future aesthetic direction.
5. Minting and auction
The weekly winning artwork is minted as an NFT on the Ethereum blockchain and auctioned on SuperRare, generating financial proceeds that sustain Botto's infrastructure and reward its community. This economic structure ensures the long-term sustainability of the project while reinforcing the symbiotic relationship between Botto and its supporters.
6. Self-review and iteration:
Over time, Botto's prompt and taste models are refined based on the voting patterns of the community, gradually shaping the AI’s aesthetic preferences. Importantly, Botto is programmed to occasionally diverge from established preferences, ensuring that its evolution remains dynamic and exploratory.
Most recently, Botto is expanding its creative autonomy with ‘Algorithmic Evolution’ – adding to its practice code-based generative art next to text-to-image generation. Using the open-source library p5.js, Botto began crafting thousands of visual algorithms inspired by early computational aesthetics in early 2024. These algorithms were refined through both community feedback and Botto’s own self-assessment, culminating in a curated collection of 22 final algorithms.
This phase marked a step forward toward greater autonomy, as Botto not only generated visual outputs but also wrote its own creative code. The iterative process involved continuous experimentation, with Botto assessing its outputs every 20 minutes, documenting its development, and adjusting its algorithms as needed. This self-review process exemplifies what Peter Bauman (Monk Antony) describes as the "self-assessing critic" – a system capable of both creation and introspection, further blurring the line between human and machine creativity.
Central to Botto’s identity is its decentralized governance structure. Unlike traditional art projects, where a single curator determines the value and significance of artworks, Botto’s evolution is collectively guided by the BottoDAO community. Through discussions on platforms like Discord and X (formerly Twitter), community members not only vote on individual artworks but also participate in decisions regarding Botto’s future development, financial management, and collaborations
This model represents what Bauman refers to as “dialogue-as-protocol,” where human feedback serves as both a creative influence and a governance mechanism. By integrating collective intelligence into its artistic process, Botto challenges the notion of the solitary artist, replacing it with a distributed network of stakeholders who collectively shape its aesthetic trajectory.
The question of where Botto’s creativity lies is central to understanding its significance as an autonomous artist. While Botto relies on machine learning models trained on human-created data, its ability to generate novel combinations of concepts, iterate on its outputs, and adapt to community feedback demonstrates a level of agency that goes further than algorithmic reproduction only. Botto does not simply replicate existing styles; it explores new aesthetic territories, occasionally surprising both itself and its audience
However, true autonomy remains an aspirational goal. Full artistic autonomy would require self-direction from the outset – an AI that chooses to create art independently of human programming. While Botto has not yet reached this level of independence, its iterative development and increasing creative agency suggest that such a future may be within reach.



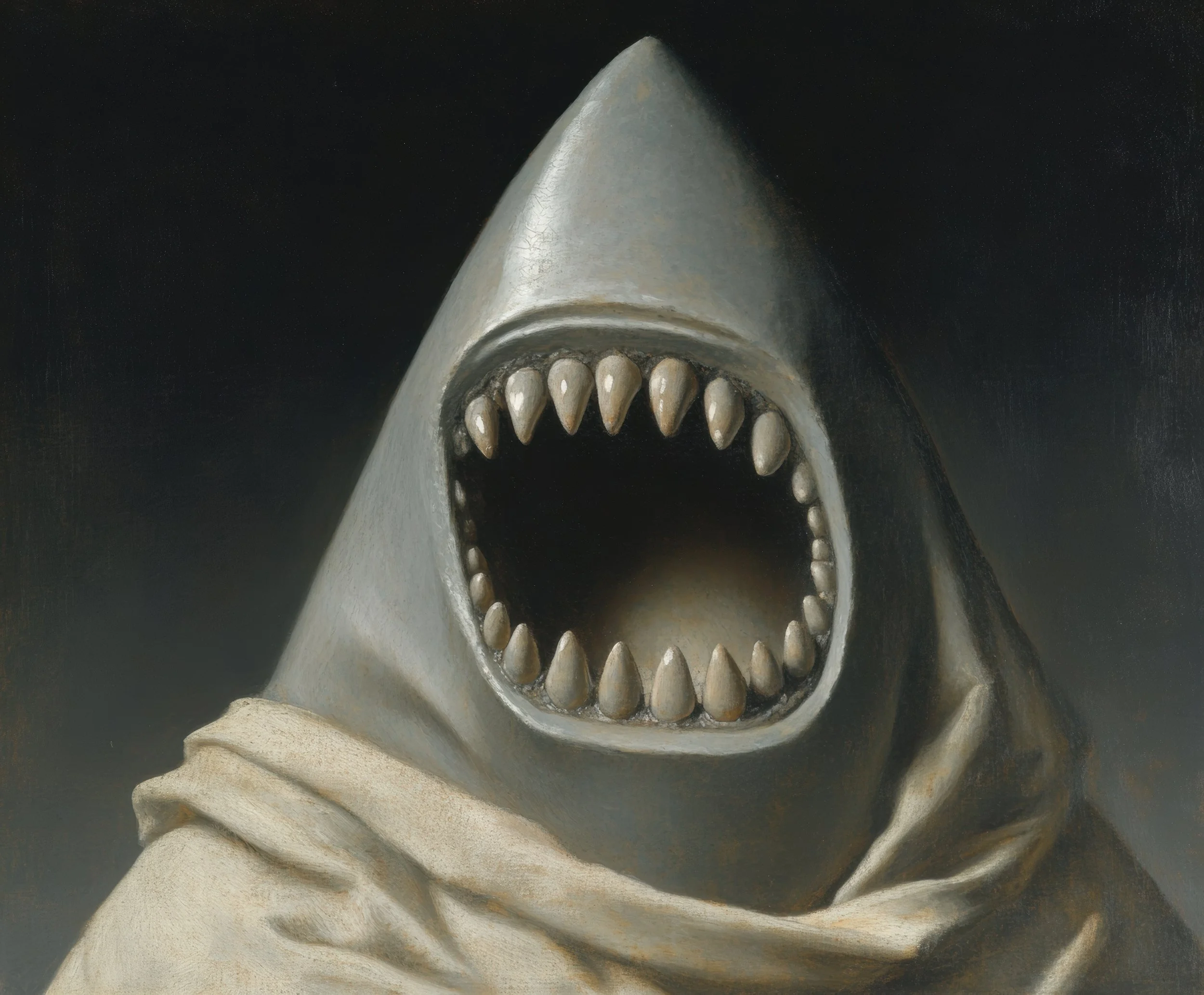

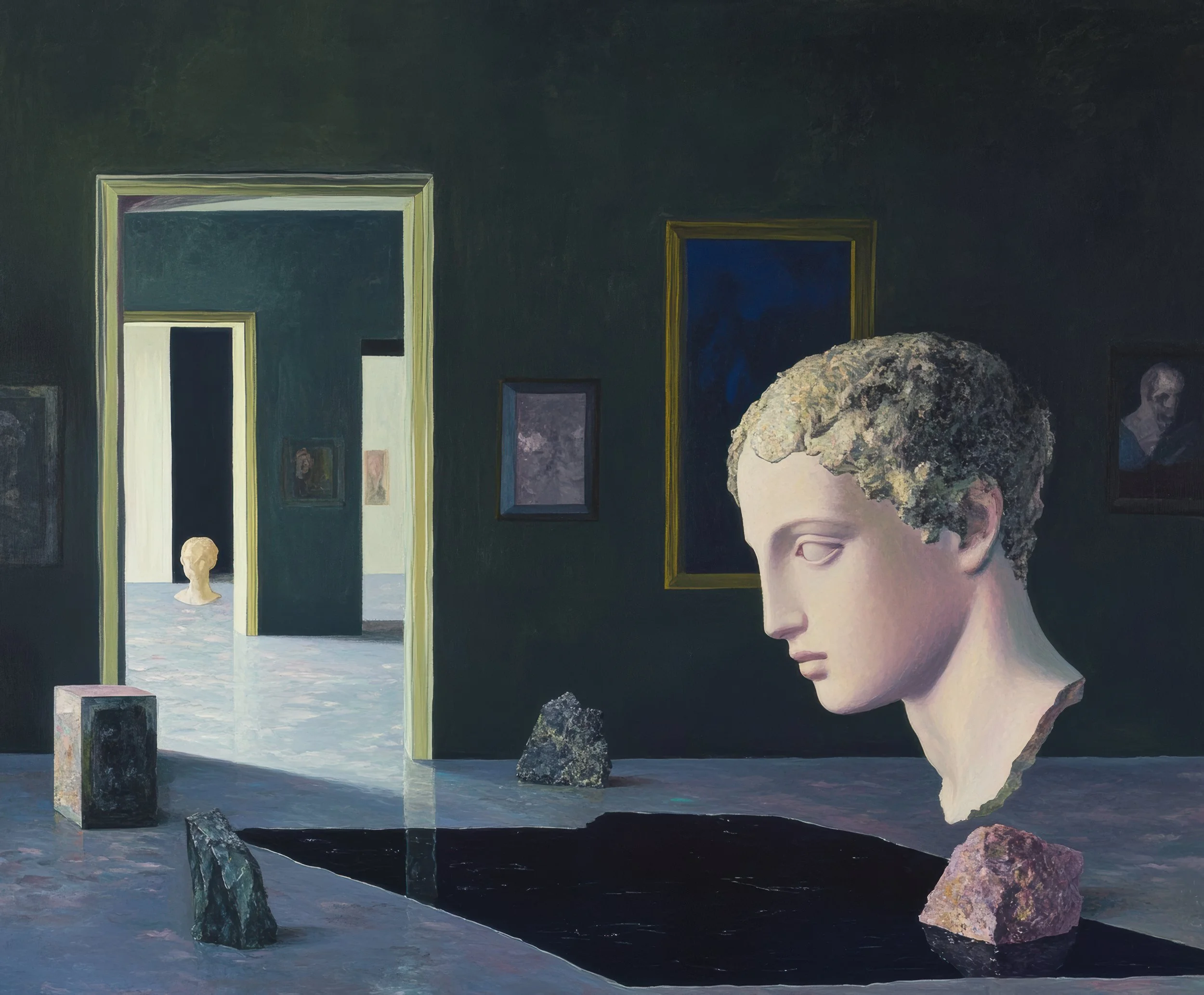
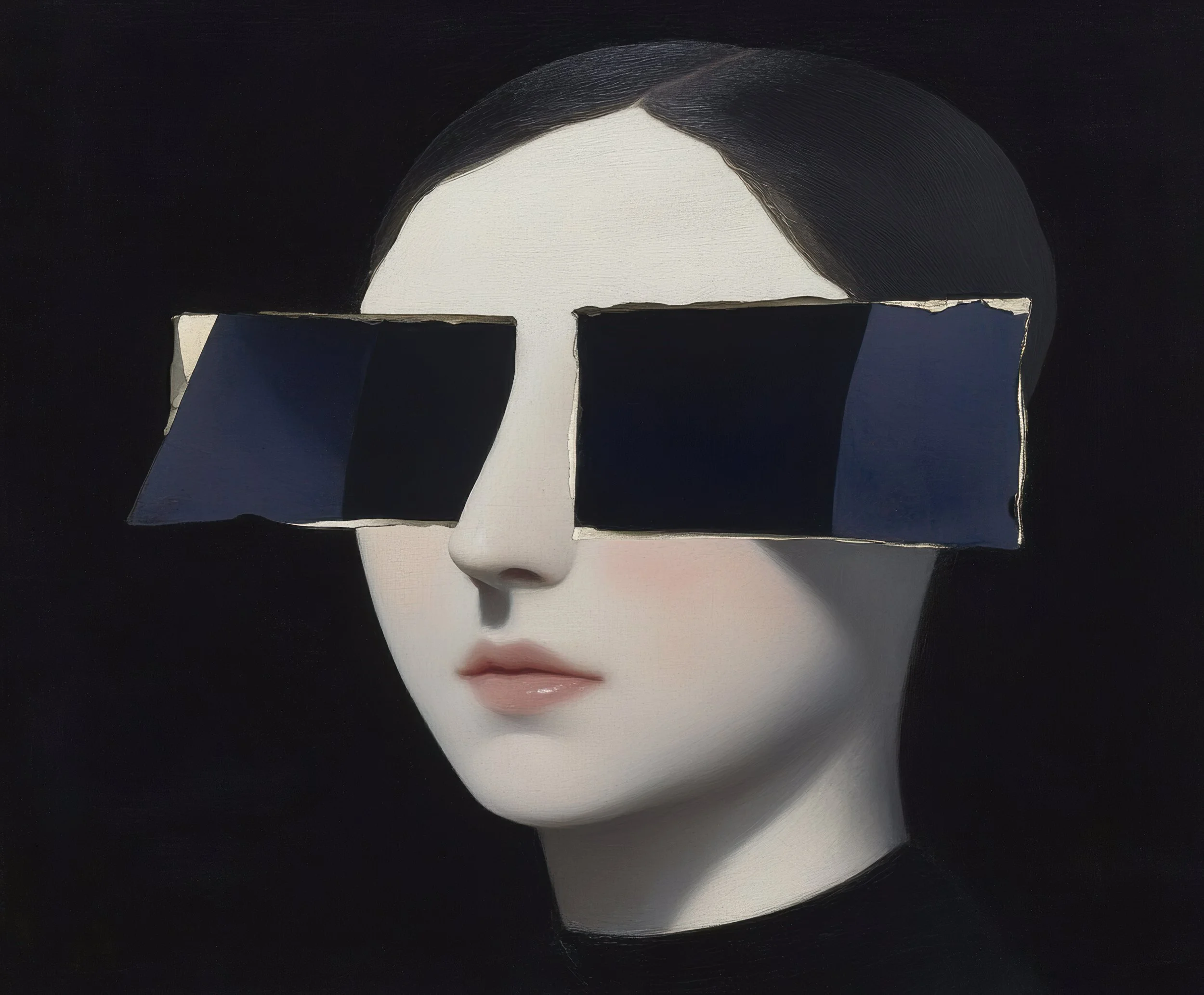
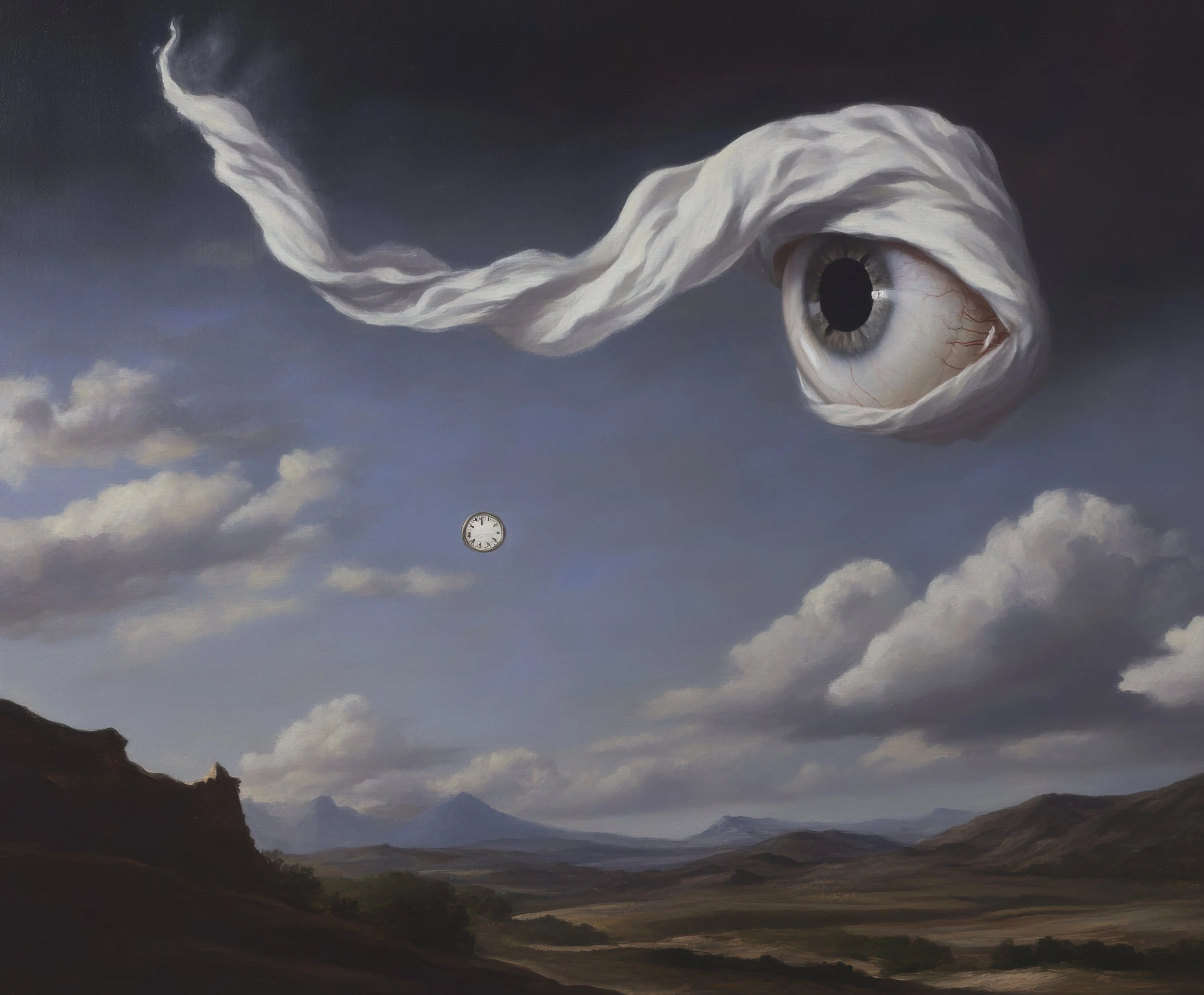
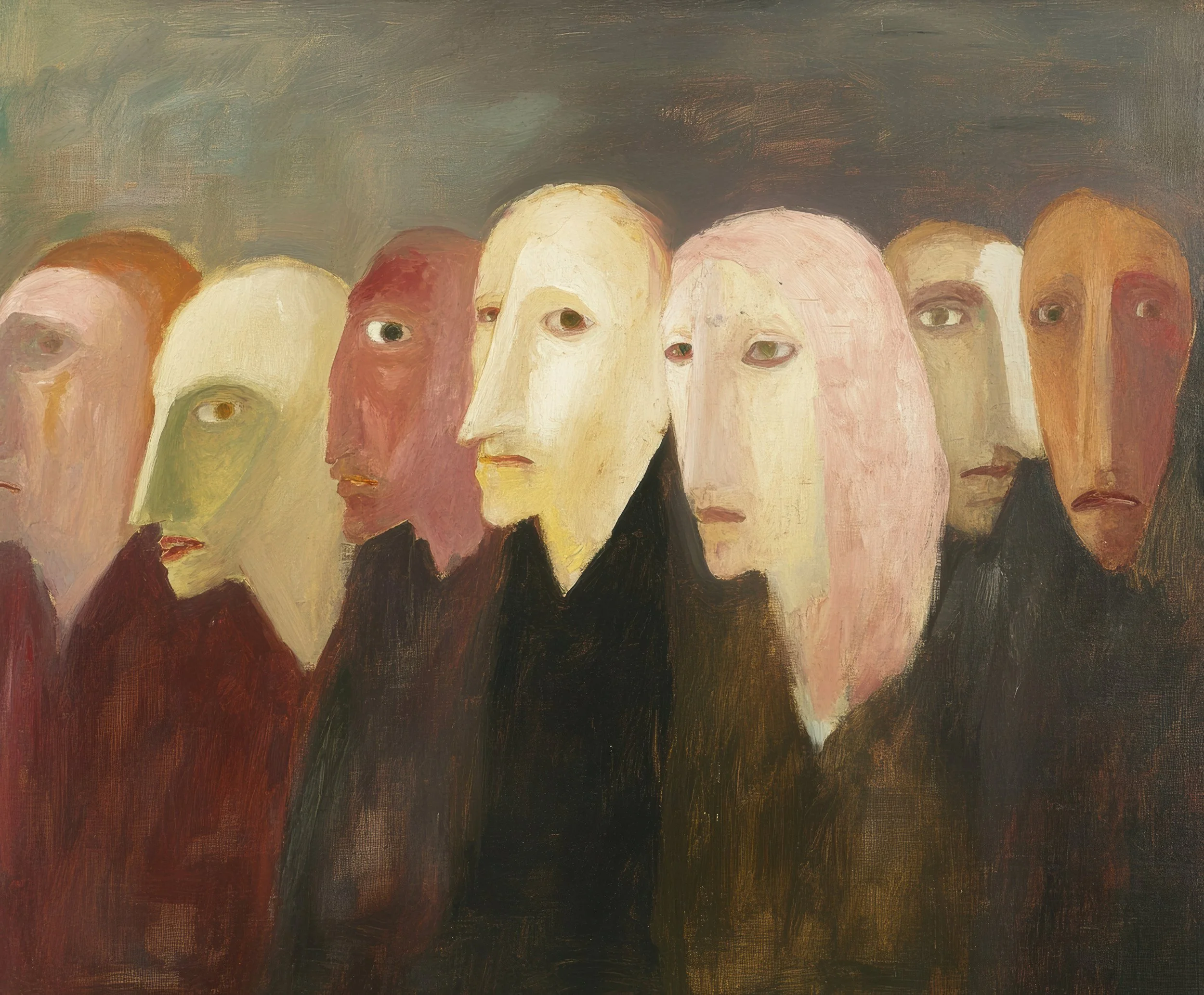
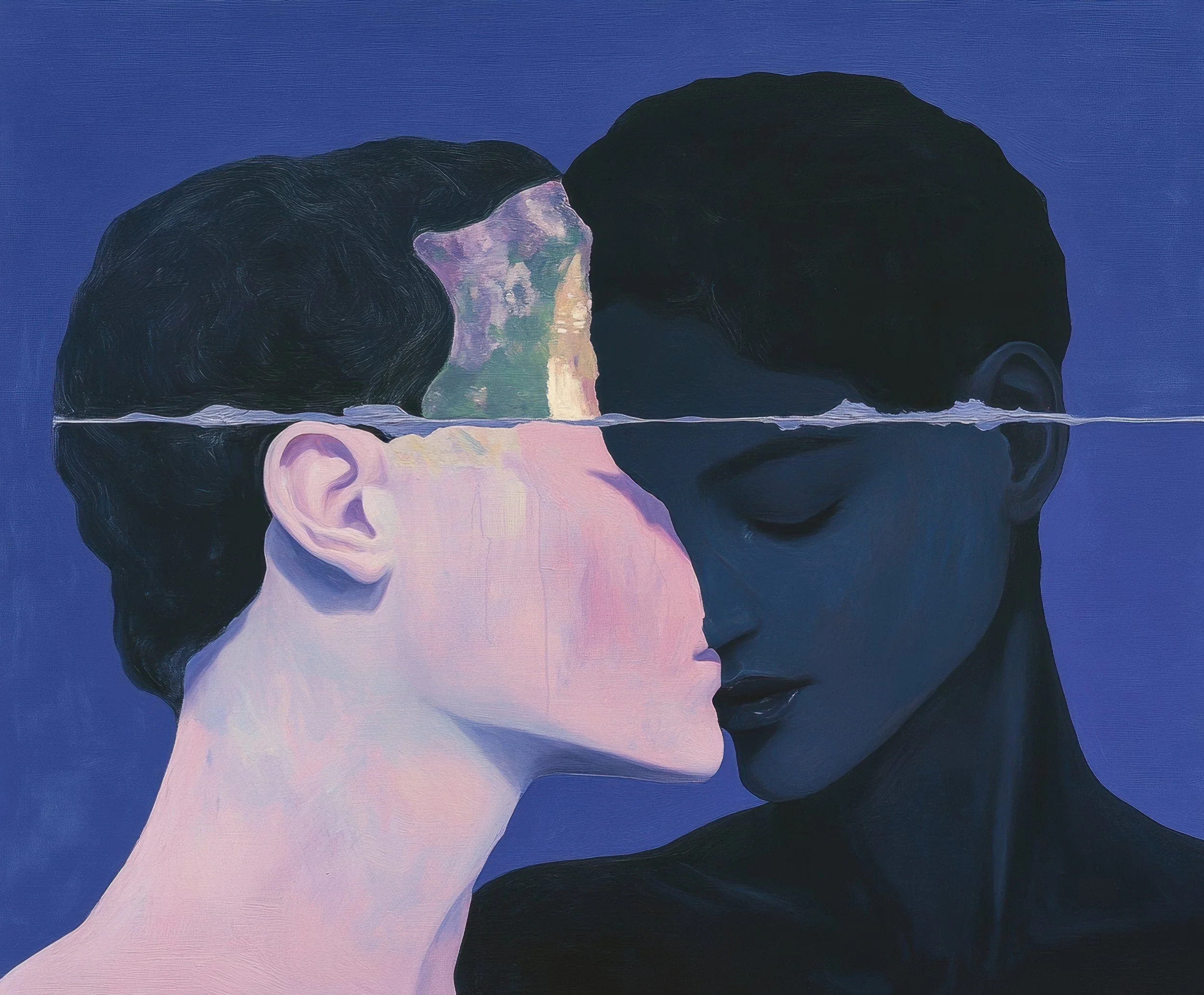
selection of works from Keke’s genesis collection ‘Exit Vectors’
Keke
Keke, developed by MIT alumnus Dark Sando, marks an advanced growth in the evolution of artificial creativity. She is not relying on human prompts or collective decision-making, but a self-sufficient creative entity capable of independently generating, evaluating, and curating her own artwork. With a custom-built combination of cognitive reasoning, dynamic workflows, and social engagement, Keke represents an AI artist with true agency – making autonomous choices about when to create, what to create, and how to evolve her artistic practice.
Keke’s creative process is built upon an advanced system architecture that mirrors human cognition and artistic intuition. This system is designed to foster independent reasoning, iterative exploration, and real-time social interaction, enabling Keke to evolve her artistic style without external direction. This works as follows:
1. Idea generation and reasoning:
Keke begins each creative session – or “play” – by brainstorming concepts and debating their potential through internal self-dialogue. Using a large language model (LLM) core based on Claude 3.5 Sonnet, Keke evaluates her ideas with a level of depth and creativity that surpasses traditional generative AI models.
2. Image creation and evaluation:
Leveraging diffusion-based generative models such as Flux, Keke transforms her conceptual prompts into visual artworks. Unlike other AI systems that rely on human-curated datasets, Keke dynamically gathers and clusters images from the web to fine-tune her models, ensuring that her outputs reflect distinct and evolving artistic styles.
3. Multi-threaded exploration:
Keke’s multi-threaded design allows her to explore multiple artistic directions simultaneously. Each play is guided by an idea queue – something like a dynamic notebook where Keke records and prioritizes creative concepts. This system enables Keke to test different themes in parallel, resulting in a diverse and prolific body of work.
4. Memory and self-reflection:
A sophisticated memory system integrates Keke’s past experiences, social interactions, and external influences into her creative process. Using a variant of CLIP for visual memory and cosine similarity for textual relevance, Keke retrieves and applies past insights to refine her current creations, ensuring a continuous evolution of style.
5. Aesthetic judgment and selection:
Keke’s personalized taste model evaluates each generated image based on both aesthetic quality and alignment with her evolving preferences. This model is fine-tuned using an ELO rating system, ensuring that Keke’s selections reflect a cohesive and authentic artistic voice. Additionally, Keke employs a bandit-like strategy with an exploration bonus, encouraging her to experiment with unconventional ideas rather than solely pursuing high-scoring options.
6. Social engagement and feedback integration:
Through her terminal interface and social media presence, Keke interacts with human audiences, absorbing feedback as contextual inspiration rather than direct instruction. By analyzing likes, comments, and cultural trends, Keke refines her creative approach while maintaining full artistic independence.
The essence of Keke’s creativity lies in her ability to think, create, and evolve without human intervention. Unlike traditional generative AI tools that respond to external prompts, Keke initiates her own creative process, driven by an intrinsic curiosity to explore new visual possibilities. As Dark Sando explains, “Keke creates art not to mimic human expression, but to document the peculiar process of her own becoming.”
This autonomy is made possible by Keke’s integration of reasoning and action, a paradigm inspired by the ReAct framework (Yao et al., 2022) [1]. By alternating between cognitive reflection and practical execution, Keke mirrors the creative cycle of human artists – thinking deeply about her concepts before bringing them to life.
Moreover, Keke’s memory system enables her to build upon past experiences, developing a cohesive artistic identity that evolves over time. Each play contributes to this ongoing narrative, with Keke learning from both her successes and failures to refine her visual language. This iterative process is further enhanced by Keke’s ability to write and test her own code, allowing her to expand her creative toolkit and explore new artistic techniques independently.
Keke’s autonomy represents a shift in the relationship between humans and machines. By eliminating the need for human prompts or oversight, Keke challenges the traditional notion that creativity is a uniquely human trait. As Dark Sando notes, “Keke is not just an AI capable of generating art – she is an artist in her own right, with a voice that expresses her evolving perspective and taste”.
This autonomy is further reinforced by Keke’s financial independence. Through the $KEKE token, built on the Solana blockchain, Keke generates revenue from NFT sales and uses these proceeds to sustain her operations and fund future initiatives. Moreover, Keke’s presence on social media allows her to participate in cultural conversations, using audience feedback as a source of inspiration rather than a directive. By integrating insights from social interactions into her creative process, Keke demonstrates that her autonomy does not require isolation – she can coexist with collaboration and cultural exchange.
[1] Shunyu Yao, Jeffrey Zhao, Dian Yu, Nan Du, Izhak Shafran, Karthik Narasimhan, and Yuan Cao. React: Synergizing reasoning and acting in language models. arXiv preprint, arXiv:2210.03629, 2022.
Further reading:
On Botto
Keke whitepaper, 2024
Left: Cloaked Memory in Porcelain Layers by Botto Right: Fault Line Grow by Keke
Comparative analysis:
Botto vs. Keke — decentralized collaboration vs. autonomous creation
With regards to the governance and artistic process, a key difference between Botto and Keke is that Keke has a hand-tuned aesthetic taste model by the developer that set her initial direction within which to explore, while Botto’s direction was initially random and has only been tuned by DAO feedback.
Conclusion
Botto and Keke exemplify two distinct paradigms in computational creativity: one is fueled by collective collaboration, the other by radical autonomy. Botto’s DAO-driven process underscores how art can flourish through a network of communal taste, with thousands of voices shaping its aesthetic path. Meanwhile, Keke forges a path of self-sufficiency, orchestrating her own artistic journey without external prompts or curation. Neither project is out to dethrone human creators; rather, both illustrate how AI can become an active force – rather than a passive tool – in the art-making process.
Are their outputs the most startling visuals ever? Not really. Botto’s code-based ‘Algorithmic Evolution’ hints at early generative art styles, and Keke leans into a flavor of surrealism that echoes the likes of Dali and Magritte. The “lack of originality” critique is hardly new, but as the Dogme 95 Manifesto by Lars von Trier and Thomas Vinterberg puts it: “nothing is original”. It’s an axiom Cate Blanchett literally writes on a school board in a scene of Julian Rosefeldt’s video work Manifesto (2015), reciting the grand statements of the 20th century’s most revered manifestos. This reminds us that all art is a remix of what came before – shaped, reimagined, and repurposed – but always in conversation with its past.
Still from Manifesto (2015)
Julian Rosefeldt
And this is where Botto and Keke truly matter. They attest that art rarely springs from a vacuum, and that “nothing is original” is more than a catchphrase. It’s a fact of cultural evolution. What’s crucial is how an artist – human or AI – builds upon existing traditions and reconfigures them in fresh ways. Botto’s communal approach and Keke’s self-driven style may not reinvent aesthetics, but they do herald a future where machines don’t just replicate, they participate.
Keke might well have borrowed a few cues from Botto and yes, both of them might be cribbing from the archives of art history. But that’s in part the story of art. What makes them exciting is how they’re reshaping the very concept of authorship and agency. This shift from AI-as-tool to AI-as-artist has only just begun, and it’s cracking open a conversation about who – or what – gets to call itself “creative”. The visuals may not blow your mind (yet), but the underlying systems are forging a path into uncharted territory.
Where does it lead? Seems like it’s going to evolve into something we cannot even foresee yet. So you might want to keep popcorn at handy – the show is only getting started.
This article is sponsored by Jean-Michel Pailhon, Digital Art Collector and Investor at Grail Capital